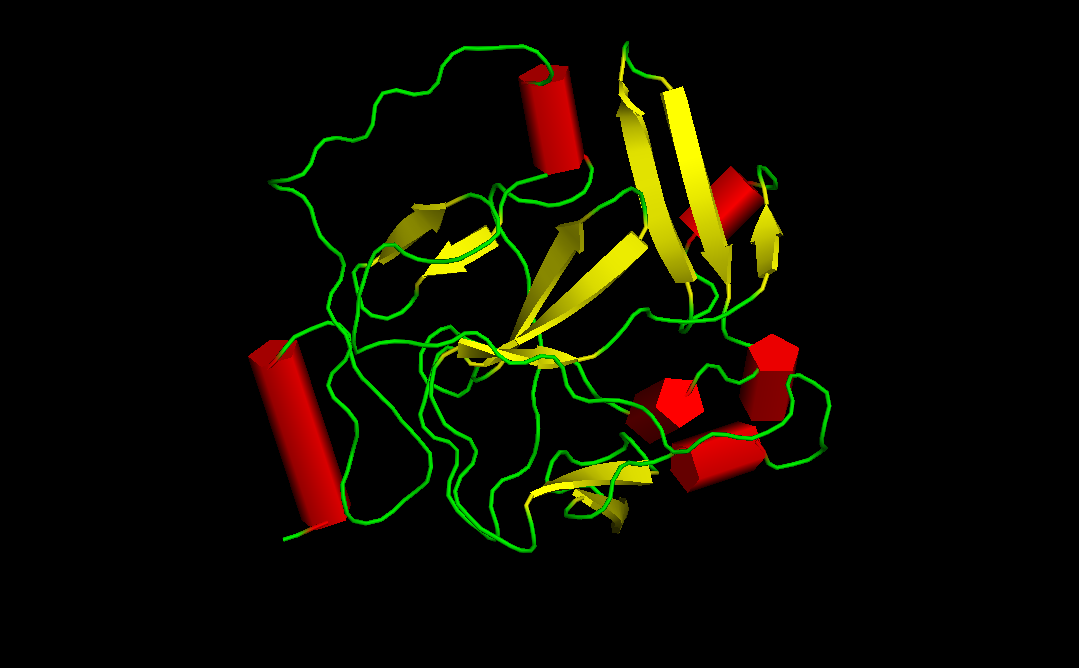
The function of a protein is impacted by its folding and shape. Knowing the 3-D conformation of a protein is essential for understanding its ability to interact with other proteins (including antibodies) or small molecules. However, deciphering protein structure has been a considerable, resource intensive, and time-consuming technical challenge, typically requiring years of work. As a result, the 3-D structures of hundreds of millions of proteins remain unknown.
Past attempts to understand protein shape have relied on costly technologies like nuclear magnetic resonance, x-ray crystallography, and cryo-electron microscopy, combined with extensive trial and error research. But now, new applications of machine learning/artificial intelligence (AI) have enabled recent “once in a generation” achievements by two separate research groups who were able to rapidly predict protein structures.
Every two years, the Protein Structure Prediction Center, a worldwide community of researchers, conducts a double-blinded, competitive assessment called the Critical Assessment of Protein Structure Prediction (CASP). The competition enables research groups to objectively test their structure prediction methods to help catalyze research and monitor progress in the field. More than 100 research groups worldwide regularly participate in CASP, the results of which set the current state-of-the-art in protein structure modeling for researchers and software users. As protein targets for scientific teams to test their structure prediction models against, CASP chooses structures that have only very recently been determined experimentally and are as yet unpublished – and so are unknown to either the competing teams or those judging the competition. The main metric used by CASP to measure the accuracy of predictions is the Global Distance Test (GDT), which represents the percentage of amino acids in the predicted structure that are within a threshold distance from their experimentally determined position in the protein.
In November 2020, CASP recognized AlphaFold2, the latest version of the AI algorithm AlphaFold developed by DeepMind (a U.K. based company owned by Alphabet, parent company of Google), as the best solution to date to the problem of predicting a protein’s 3-D structure based just its amino acid sequence. AlphaFold2 achieved a median score of 92.4 GDT across all protein targets, and, for the most difficult proteins, a median score of 87.0 GDT. For reference, a score of around 90 GDT for a predicted amino acid position is considered competitive with the value obtained experimentally. DeepMind trained AlphaFold2 on data from 170,000 publicly available protein structures plus a large database of protein sequences whose 3-D structures remained unknown.
In July 2021, DeepMind published its work and the source code for AlphaFold2 in Nature.
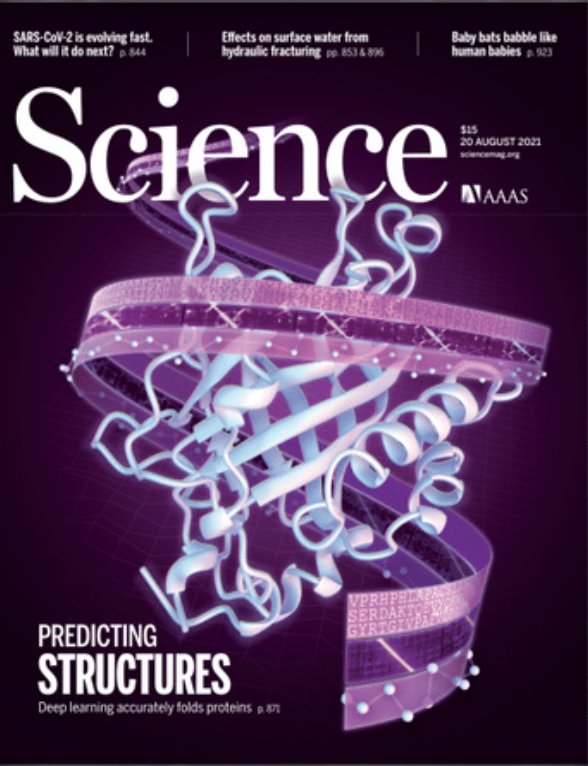
Inspired by DeepMind’s November CASP win, a second international research team led by the University of Washington, Seattle developed their own protein prediction algorithm and in July, they published their work in Science. This team’s algorithm, RoseTTAFold uses a three-track neural network to simultaneously consider patterns in protein sequences, how amino acids interact, and the possible 3-D structures the protein may form to predict the optimum protein conformation.
While AlphaFold2’s predictions were somewhat more accurate, the RoseTTAFold predictions were nearly as good and performed better when predicting certain types of protein structures. Moreover, while AlphaFold2 addressed only single protein structures, RoseTTAFold was able to predict how multiple proteins may arrange into multi-protein complexes (i.e., quaternary structure). In addition, both algorithms solved such structures with considerable speed. Compared to experimental methods, which required months to years of work, both AI-based models returned their predictions within minutes to hours.
In the weeks following their respective scientific publications, both the DeepMind and University of Washington teams made their data, methodology, and source codes freely available to scientists worldwide. Moreover, in collaboration with the European Molecule Biology Lab’s European Bioinformatics Institute, DeepMind has launched the AlphaFold Protein Structure Database, freely offering high-quality shape predictions for every human protein to the scientific community, as well as the proteins of 20 other organisms (e.g., E. coli, yeast, fruit fly, mouse) commonly used in scientific research.
Experts believe this new ability to rapidly decipher protein folding is likely to open up new research avenues with applications in pharma R&D, which until now has been limited by scientists’ inability to determine protein structures. This advance is expected not only to accelerate researchers’ ability to understand diseases and develop drugs with greater specificity, but also to catalyze advances outside of medicine, such as developing new industrial enzymes to break down plastic wastes or other pollutants, or even capture carbon from the atmosphere.